Andreas Groll, with his successful prediction of the European Championship 2012 already experienced in this field, and Gunther Schauberger did set out to predict the 2014 world cup champion based on statistical modeling techniques and R.
A bit surprisingly, Germany is estimated with highest probability of winning the trophy (28.80%), exceeding Brazil’s probability (the favorite according to most bookmakers) only marginally (27.65%). You can find all estimated probabilities compared to the respective odds from a German bookmaker in the graphic on their homepage (http://www.statistik.lmu.de/~schauberger/research.html), together with the most likely world cup evolution simulated from their model. The evolution also shows the neck-and-neck race between Germany and Brazil: they are predicted to meet each other in the semi-finals, where Germany’s probability of winning the game is a hair’s breadth above 50%. Although there does not exist a detailed technical report on the results yet, you still can get some insight into the model as well as the data used through a preliminary summary pdf on their homepage (http://www.statistik.lmu.de/~schauberger/WMGrollSchauberger.pdf).

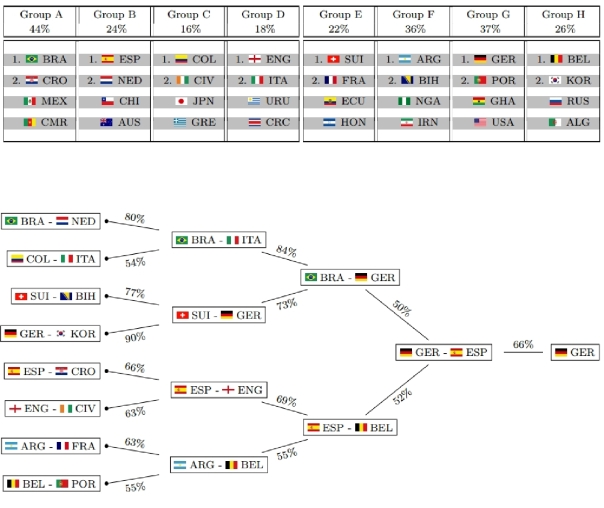
Last week, I had the chance to witness a presentation of their preliminary results at the research seminar of the Department of Statistics (a home game for both), where they presented an already solid first predictive model based on the glmmLasso R package. However, continuously refining the model to the last minute, it now did receive its final touch, as they published the predictions at their homepage.
As they pointed out, statistical prediction of the world cup champion builds on two separate components. First, you need to reveal the individual team strengths – “who is best?”, so to speak. Afterwards, you need to simulate the evolution of the championship, given the actual world cup group drawings. This accounts for the fact that even quite capable teams might still miss the playoffs, given that they were drawn into a group of hard competitors.
Revealing the team strength turns out to be the hard part of the problem, as there exists no simple linear ranking for teams from best to worst. A team that might win more games on average still could have its problems with a less successful team, simply because they fail to adjust to the opponents style of play. In other words: tough tacklings and fouls could be the skillful players’ death.
Hence, Andreas Groll and Gunther Schauberger chose a quite complex approach: they determine the odds of a game through the number of goals that each team is going to score. Thereby, again, the likelihood of scoring more goals than the opponent depends on much more than just a single measure of team strength. First, the number of own goals depends on both teams’ capabilities: your own, as well as that of your opponent. As mediocre team, you score more goals against underdogs than against title aspirants. And second, your strength might be unevenly distributed across different parts of the team: your defense might be more competitive than your offensive or the other way round. As an example, although Switzerland’s overall strength is not within reach to the most capable teams, their defense during the last world cup still was such insurmountable that they did not receive a single goal (penalty shooting excluded).
The first preliminary model shown in the research seminar did seem to do a great job in revealing overall team strength already. However, subtleties as the differentiation between offensive and defense were not included yet. The final version, in contrast, now even allows such a distinction. Furthermore, the previous random effects model did build its prediction mainly on the data of past results itself, referring to explanatory co-variates only minor. Although this in no way indicates any prediction inaccuracies, one still would prefer models to have a more interpretable structure: not only knowing WHICH teams are best, but also WHY. Hence, instead of directly estimating team strength from past results, it is much nicer to have them estimated as a result of two components: the strength predicted by co-variates like FIFA rank, odds, etc, plus a small deviation found by the model through past results itself. As a side effect, the model should also become more robust against structural breaks this way: a team with very poor performance in the past now still could be classified as good if indicators of current team strength (like the number of champions league players or the current odds) hint to higher team strength.
Building on explanatory variables, however, the efficient identification of variables with true explanatory power out of a large set of possible variables is the real challenge. Hence, instead of throwing in all variables at once, their regularization approach allows to gradually extend the model by incorporating the variable with best explanatory power among all not yet included variables. This variable selection seems to me to be the big selling point of their statistical model, and with both Andreas Groll and Gunther Schauberger having prior publications in the field already, they most likely should know what they are doing.
From what I have heard, I think we can expect a technical report with more detailed analysis within the next weeks. I’m already quite excited about getting to know how large the estimated distinction between offensive and defense actually turns out to be in their model. Hopefully, we will get these results at a still early stage of the running world cup. The problem, however, is that some explanatory variables within their model could only be determined completely when all the team’s actual squads were known, and hence they could start their analysis only very shortly prior to the beginning of the world cup. Although this obviously caused some delay for their analysis, this made sure that even possible changes of team strength due to injuries could be taken into account. I am quite sure, however, that they will catch up on the delay during the next days, as I think that they are quite big football fans themselves, and hence are most likely as curious about the detailed results as we are…
Source: http://www.r-bloggers.com/prediction-model-for-the-fifa-world-cup-2014/
I didn't quite understand the statistical model hmm..
ReplyDelete--
World Cup Bet
Well, applied the previous dataset and used statistical and probabilistic methods to model the possibilities, but yes, it is not really correct.
ReplyDelete